Ethical challenges in AI-driven diagnosis and healthcare
By Desmond Cheung & Roderick Van Den Bergh
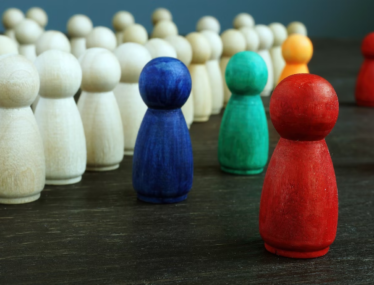
The use of artificial intelligence (AI) to aid medical diagnosis or rationalise healthcare raises similar ethical challenges as applications of AI in other industries, bias and discrimination among them. A prescription of selective forgetfulness for the AI may be a remedy, argue Roderick van den Bergh and Desmond Cheung.
AI has transformed industries such as online advertising and computer games. It also has great promise for transforming healthcare by optimising the delivery of services (as well as unlocking new ones), reducing healthcare professional workloads, and eventually becoming integrated across healthcare value chains [1, 2]. But stakes here are higher than in non-healthcare industries. How do we address concerns of social bias and deviation from societal values in order to reap the full benefits of AI technology for healthcare?
When medical applications of AI are evaluated, a heavy emphasis is often placed on its quantitative performance against humans, such as how the AI fared against “a panel of physicians” and on statistical metrics.
For example, a recent international study of using AI to screen for breast cancer emphasized that the AI “surpassed clinical specialists” on metrics such as false negatives, false positives and achieved “non-inferior performance” compared to the standard clinical workflow for breast X-rays [3].
Indeed, the emphasis on quantitative metrics is equally common for studies involving other types of medical data, such as ECGs or electronic health records, and is often exemplified in AI competitions where winners are judged through the optimization of certain metrics.
While such metrics undeniably provide an objective and measurable evaluation of the AI’s performance on a dataset, and show the technological strides made in the field recently, an over-reliance on metrics in medical AI may actually deepen hidden biases embedded within the dataset and exaggerate unequal health outcomes based on socio-economic factors.
Bias and discrimination within AI is well-documented: sometimes it is due to a lack of good, clean or balanced data, as has been seen recently with SARS-COV-19 detection models, some of which have been based on self-reported data [4]; and sometimes it arises because existing algorithms for interpreting data are deficient, as seen in the recent failures of the Twitter facial recognition model, which included white faces in photos on mobile screens more frequently than black faces [5].
In healthcare, a serious additional concern is that even an AI model based on robust data and algorithms and with good statistical performance may have learnt to perpetuate existing social trends and biases, leading to sub-optimal quality of care or health outcomes for individuals belonging to certain socio-economic groups.
An over-reliance on metrics in medical AI may deepen hidden biases embedded within the dataset and exaggerate unequal health outcomes based on socio-economic factors.
In this vein, a recent study highlighted that an algorithm used by US health insurers to predict and allocate resources based on how ill a patient is had used medical billing records as training data and as a proxy for health [6]. This caused the model to absorb that people self-identifying as black in the past received less treatment, but for a variety of socio-economic reasons rather than lack of need.
This algorithm, when presented with two patients that self-identify as black and white, would allocate more resources and treatment to the white than to the black patient. And it would do so even though the black patient would be more unwell and benefit to a greater extent from healthcare – contrary to societal values.
When developing medical applications of AI we must therefore be careful how we use data and recognise that context is everything. We must recognise that data dis-aggregation may be important in some contexts, such as heart attacks where symptoms differ between men and women, whilst in other contexts we must consider a prescription of “selective forgetfulness” by hiding information from the network, such as in the insurance example above.
The purpose of technology in healthcare is to improve outcomes and quality of care, and we need to remember that this may not be strictly aligned with the statistical performance of an AI model. Medical applications of AI should be developed by a multidisciplinary team who are able to tease apart causation and correlation within the data and prevent the AI model recreating correlation where it is detrimental.
Crucially, we need to understand that incorporating values into AI may result in a model which has a lower statistical performance but results in better outcomes for patients and society. Only through close collaboration between society, medical AI developers and regulators will we be able to align and bring the full benefit of this technology to people’s lives.
Digital Health | Medical Devices | em. Digital Therapeutic for BPD case study | Healthcare Blogs | Healthcare Case Studies