Generative AI is already having a transformative effect on many aspects of business, including common functions such as admin, sales and marketing. These advances have mostly been powered by foundation models, which are machine-learning models trained on large amounts of generalised data. These models are useful because they are very adaptable, and the results are familiar to us in the form of text and image generators, and digital assistants able to understand and converse in natural language.
As we improve our understanding of how to use foundation models, attention is now turning to more specialist tasks – including the development and operation of highly complex products. This is part of what we help our clients do at TTP, and one aspect of our work is developing ‘digital twins’ to reduce the reliance on physical prototyping and, in particular, having to make untested changes on an actual system. So with the applications of foundation models continuing to expand, we asked ourselves a question – could they help us to streamline the development of digital twins?
What is a digital twin?
But first, a bit of background. A digital twin is essentially an in silico model of a physical system that aims to accurately mirror its operation. This model can do this at various levels, from a single component or group of components, through to a complete system, or even an entire process. Importantly, because digital twins can encode the actual behaviour of the system (in contrast to a static physics-based model, for example), it is relatively straightforward to refine them as more data is gathered on how the system responds to changes. This means that their usefulness continues to improve over time.
Digital twins have value at two stages of a product’s lifecycle:
- Product development: When developing complex products, it’s often necessary to consider a range of possible concepts in order to decide on the best design. Digital twins allow product development to remain in the ‘virtual world’ for longer, and so reduce the cost of prototype development and testing and also increase the scope of what you can test.
- Product operation: Once the product is in use, decisions need to be made on how to modify its operation in response to changing circumstances. Digital twins can take the uncertainty out of such decisions, avoiding having to tinker with a live system, and the cost implications of getting it wrong.
Using digital twins in industry
Digital twins are already being used in various scenarios to mitigate risk and speed up decision-making. A good example is Formula 1 racing, where ‘driver in the loop’ simulators have allowed innovations to be rigorously tested, avoiding wasting time and money in the production of parts that don’t end up performing as needed.
Likewise, once the car reaches the track, digital twins can be used to quickly test a variety of settings (such as suspension stiffness, ride height and wheel camber) in response to track layout and driving conditions encountered in practice. Feedback can be provided between practice sessions, improving the model and through use of the simulator to recommend changes in set-up, ultimately making the difference between winning and losing on race day.
Digital twins have also have the potential to optimize the control of various types of electrolysers in hydrogen-generating plants powered by renewable electricity from intermittent sources such as solar and wind. This would not only improve plant performance but also extend the lifetime of the components.
And speaking of renewable technologies, at TTP we helped our client HSLU develop a “skeleton” digital twin for different designs of algal bioreactor. This helped them to improve the design and fine-tune the operating conditions to either capture carbon dioxide from the atmosphere, or manufacture high-value chemicals such as pharmaceuticals.
Overcoming barriers using foundation models
But if digital twins are so useful, why aren’t we seeing them everywhere? The main reason is that building a digital twin depends upon a deep knowledge of the system being modelled, including access to sensing data. In addition, using software expertise to integrate all this information into a functioning model is a niche skill, and the whole process is fundamentally time-consuming. All of this has meant that developing digital twins has only been cost-effective where high-stakes decisions need to be made, and where the case for business investment is clearest.
But all that could change if we can harness foundation models to give us a head-start. Studies in our own AI lab have indicated that, in many cases, foundation models do an excellent job of providing a first-pass or “seed” digital twin that is quite workable. And importantly, because we’re using AI, this part of the development process is completed much more quickly than was possible previously. This means that the knowledge of experts – whether from the software or product-engineering side – can be directed towards refining the digital twin, rather than laboriously building it from the ground up.
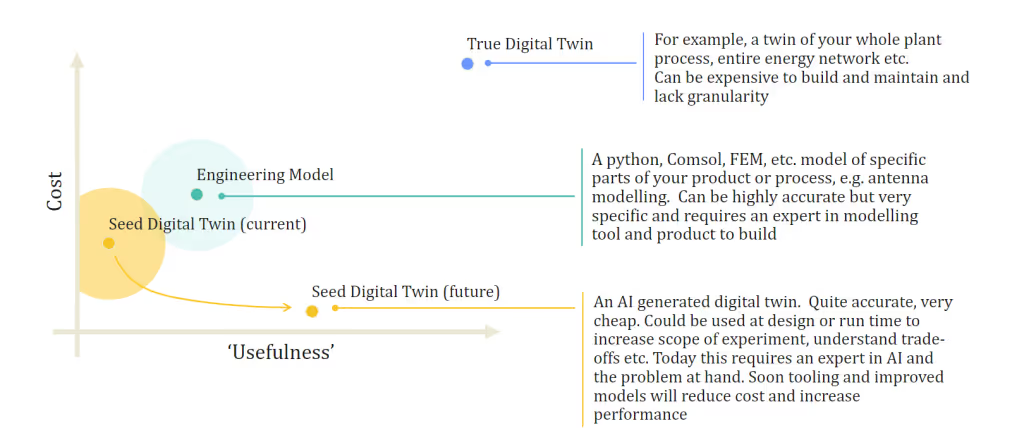
At the same time, the continuing increase in the availability of high-performance, low-cost sensors that are small, light, and have low power requirements makes it possible to achieve a much greater understanding of complex physical products through sensor data. This allows us to generate the fine-grained information that is needed to inform the development of accurate digital twins for complex devices.
Combining the best of human and AI capabilities
In conclusion, the insights we’ve gained through our studies show that foundation AI models, by streamlining the development of ‘seed’ digital twins, could remove the barriers of complexity and cost that currently stand in the way of wider take-up.
This opens up the prospect of developing digital twins for products in cases where it was previously difficult to make a business case for investment. This applies both to applications where the stakes are not as high, but also to situations where numerous individual components of a more complex system need to be fine-tuned e.g., in a hierarchical network of assets such as an energy distribution network. The result will ultimately be better-optimised products and systems developed within a shorter timeframe, and performing to a higher standard in everyday operation.
Nevertheless, expert human input will remain essential, both during ‘refinement’ of the digital twin, as well as in validating its output. But what’s clear is that only by combining human expertise with AI-based tools, we’ll be able to unlock the true potential of digital twins, and so make this useful technology more accessible across a wider range of applications.
In future TTP Insight articles, I’ll be taking a closer look at how AI tools can be applied to digital twin development in different markets and industries. In the meantime, if you’d like to have a chat about what digital twins could do for your product or process, please get in touch.