Fashion or Future? AI as part of the business transformation toolkit
By Dr Simon Shakespeare
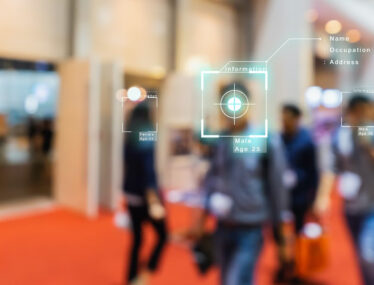
Artificial Intelligence could be the solution to all our woes or the most over-hyped technology of our age. Even defining AI can seem problematical, given that we have trouble defining what regular intelligence is. Does that mean AI is over-rated, or just that our expectations of what it can do are over-inflated? How does AI really fit into the toolkit for business transformation?
The first piece in the puzzle is to dispel the notion that all AI development is about creating thinking machines that can replace thinking humans. Perhaps general artificial intelligence is coming, but this is a subject for a very small number of academic researchers in the area. Most commercial development is for specific applications that learn patterns from lots of data. Unfortunately, the term ‘AI’ has subsumed all forms of machine learning under one umbrella, which has misled many into believing that general AI is about to arrive and replace us all.
Application-specific AI is an extension of statistical data mining combined with old-fashioned machine learning, but with vastly more data and computer processing power. In industry this might be combined with automation and robots, but again this trend has been ongoing since the beginning of the industrial revolution. These systems replace tasks that are repetitive and predictable, but not ones requiring insight, creativity or cognition. AI’s ability to classify data accurately is impressive, often at a level that matches or exceeds human capability. Indeed, in the ImageNet competition, the machine classifier surpassed human error rates of 5.1% when classifying images in 2015.
Before looking at the technology in the black box, it’s reasonable to ask if it is over-hyped or genuinely revolutionary. Gartner believe that Deep Learning and many other Neural Network techniques are reaching ‘peak AI’ hype levels [1], but this may simply reflect the speculative reporting of the technology by journalists in eye-catching articles. Investment research, such as that reported by Tata and McKinsey for example, does not show a peak, but a healthy growth in investment year-on-year [2, 3]. Application-specific machine learning is being used successfully, and profitably, as companies such as Amazon, Alphabet, Facebook and Apple will attest. This is not to say that AI is only for large tech corporations, but those investing heavily in the area are doing so as it gives them a competitive edge, new service offerings and a route to profit.
As part of a business toolbox, machine learning can be, and has been used for many modest projects by companies who are not billion-dollar tech-leviathans. As an example, a machine vision system developed as early as 2005 for recognising traffic signs using traditional image processing could now be developed much faster and to a higher accuracy, using modern techniques such as convolutional neural networks. Similarly, technologies for recognising human faces, emotion and pose exist now in a way that was unthinkable just a few years ago. The democratisation of machine learning tools and the open-source nature of developments in the area has enabled the power of the technology to become more widely spread, and available to all.
For many companies, the lack of internal skilled resource, legacy infrastructure, data availability and budget may hamper the introduction of AI technology. Yet, they need not do so. In most contexts, it makes sense to manage the risks of technology introduction through investigative studies and small trials with appropriate external guidance. Taking a stepped approach to a new development helps define the requirements and set achievable milestones. Studies of AI deployment by Intel and Harvard Business Review also back up a pragmatic and staged approach to both investigating the technology and then introducing it into an existing business [4, 5]. Improving a process may often be more acceptable than a transformative change that might disrupt all aspects of the business. Where AI has been transformative, the most successful approaches are where AI is not just bolted on but has been integrated into the business.
In summary, although AI has become over-hyped by journalists, there is much evidence to show that, when implemented correctly and with the correct commercial focus, AI can make a positive contribution. For some companies the introduction of AI is transformative, changing the fundamental business model, but for many more the introduction can be incremental and more modest. Lacking internal resource in AI should not limit ambition. There are many opportunities and many new tools for building capability where a value proposition exists as part of an AI-enabled future, rather than as a passing fashion.
Telecoms | Industrials | Telecoms Blogs | Industrials Blogs | Telecoms Case Studies | Industrials Case Studies